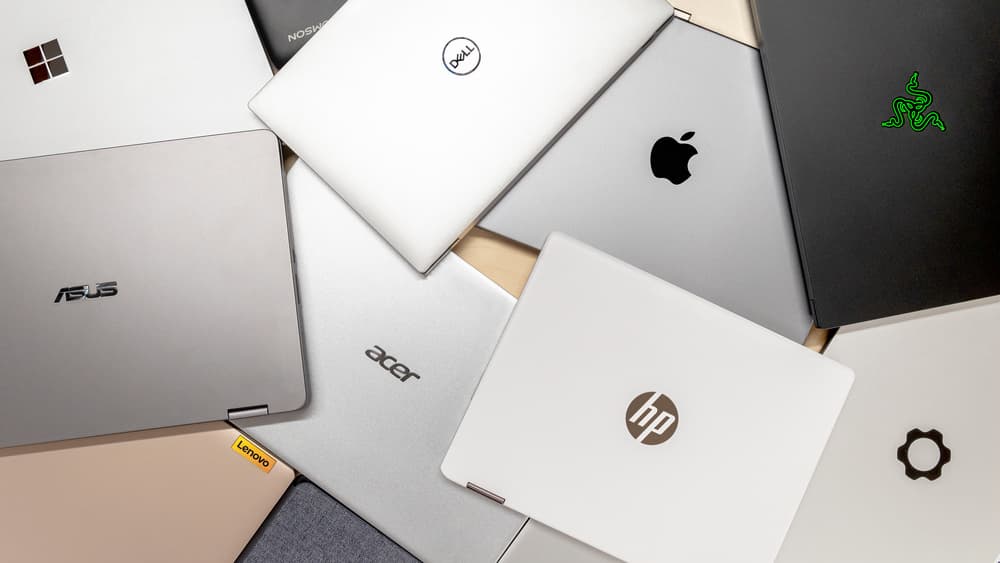
Predictive Analysis of Laptop Ratings: Leveraging Machine Learning for Market Insights
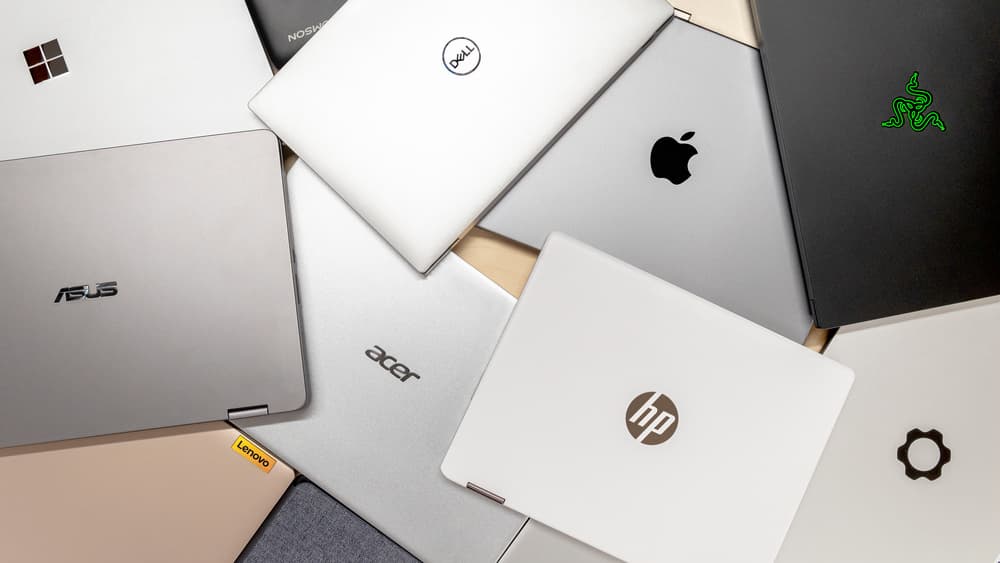
Project Summary
In my project, I analyzed Flipkart's extensive laptop inventory using machine learning to predict user ratings from a 2023 dataset obtained from Kaggle. The data was meticulously cleaned and transformed, focusing on key specifications like brand, processor, operating system, and price. This preparation was essential for the effectiveness of my predictive models.
I used Linear Regression and K-Nearest Neighbors (KNN) models to understand how various laptop features correlate with user ratings. Through thorough testing and feature optimization, I aimed to enhance the prediction accuracy, allowing me to compare the efficacy of these models in understanding my dataset's complex patterns.
The results revealed that the KNN model was more effective than Linear Regression, suggesting complex relationships between laptop features and user ratings. This insight is valuable for laptop manufacturers and retailers for strategizing product development and marketing. Furthermore, the project highlighted the potential of machine learning in analyzing consumer electronics markets, paving the way for more detailed future studies.